Today, performance marketers have a huge variety of measurement models and methods at their disposal: ID attribution, SKAdNetwork (SKAN), media mix modeling (MMM), and incrementality, to name a few. Let’s dig into the options to discover their unique benefits, pitfalls, interoperability, and potential to achieve your strategic goals. We sat down with Maor Sadra, CEO of INCRMNTAL, to decipher the diverse ad tech measurement frameworks.
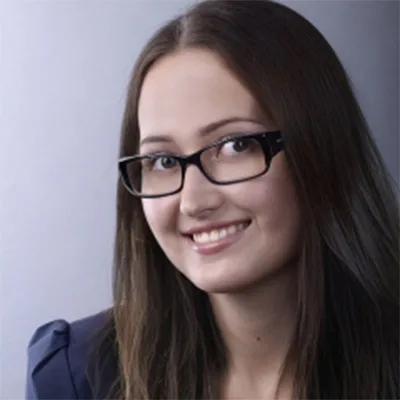
Should marketers consider adopting multiple measurement approaches?
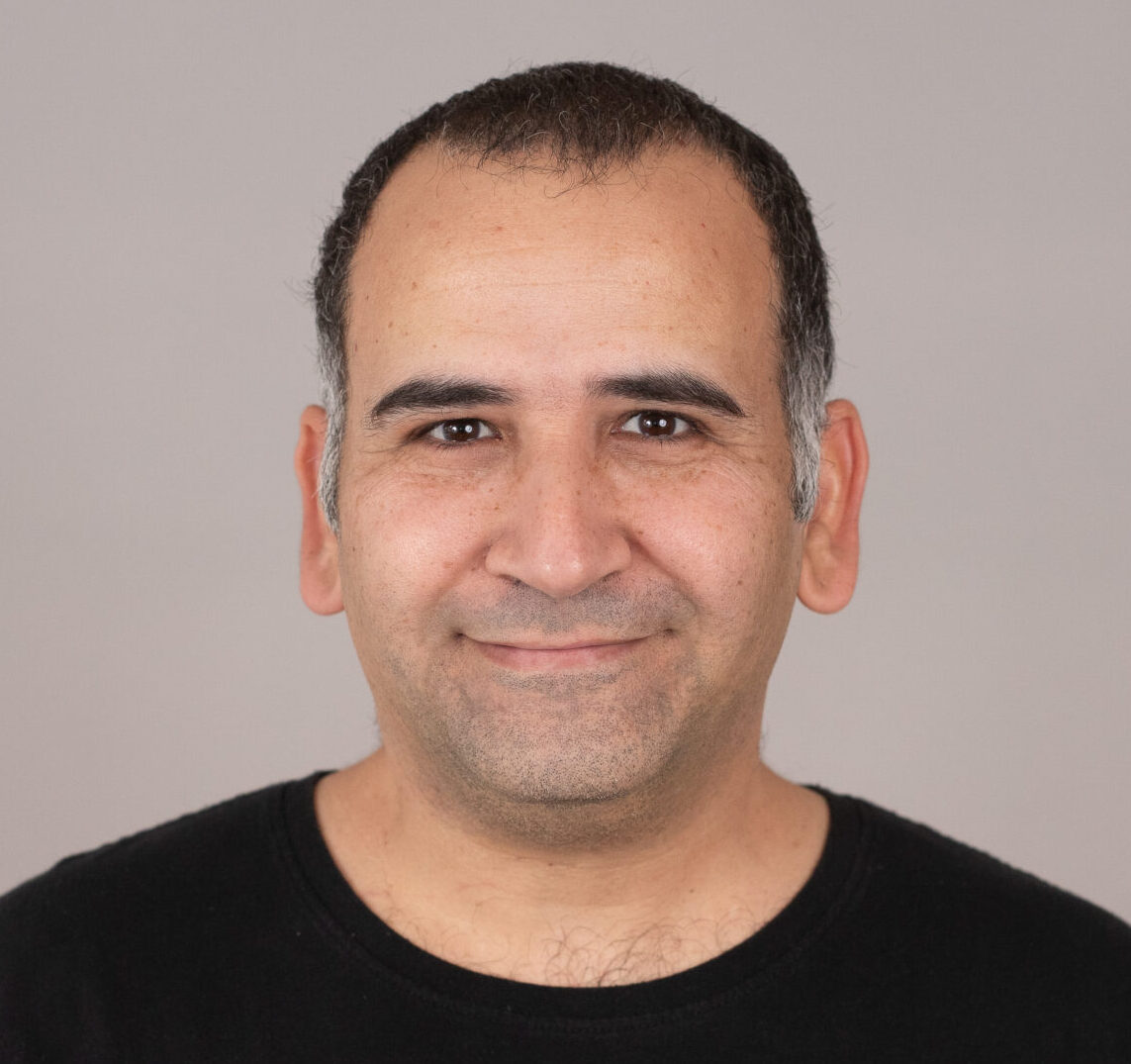
Yes, 100%. The multifaceted strategy addresses different needs and scenarios more effectively.
Deterministic methods, like last-touch attribution (whether through fingerprinting, SKAdNetwork/AttributionKit), provide critical insights. They tell you who “scored the goal” and offer near real-time data, crucial for optimizing campaigns based on immediate performance. For instance, if a red banner has a 20% conversion rate compared to a blue banner’s 5%, deterministic attribution quickly identifies the more effective creative without needing further analysis.
However, for advertisers with substantial budgets, relying solely on deterministic methods isn’t sufficient. Different methodologies serve distinct purposes.
Last-Touch Attribution: ideal for real-time optimization and understanding which creatives perform better. It’s irrefutable and necessary for immediate decisions.
Incrementality: crucial for evaluating the impact of campaigns that aren’t easily trackable through clicks, like CTV campaigns. It provides near real-time insights (e.g., a week after a campaign starts) on what is working, guiding adjustments and optimizations without pausing campaigns or conducting A/B tests.
Media Mix Modeling (MMM): offers a holistic view of long-term brand effects, suitable for high-level forecasting and scenario planning. It operates on a longer timeline, such as monthly or quarterly, and helps in understanding the broader impact of campaigns.
For example, an advertiser launching a new TV campaign can’t rely on attribution methods due to the non-clickable nature of TV ads. Incrementality measurement will provide a good indication of the campaign’s impact shortly after launch, guiding real-time decisions on spending and adjustments. MMM, on the other hand, will help understand the long-term brand effects of the campaign but won’t be useful for day-to-day optimization.
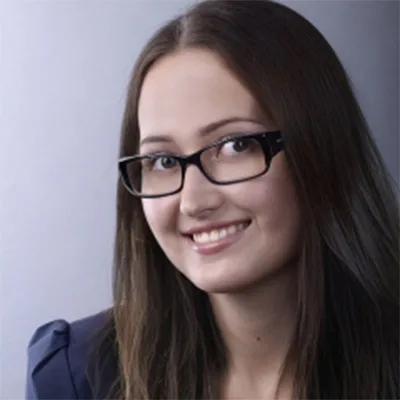
Can you give us some successful examples of using multiple measurements approaches?
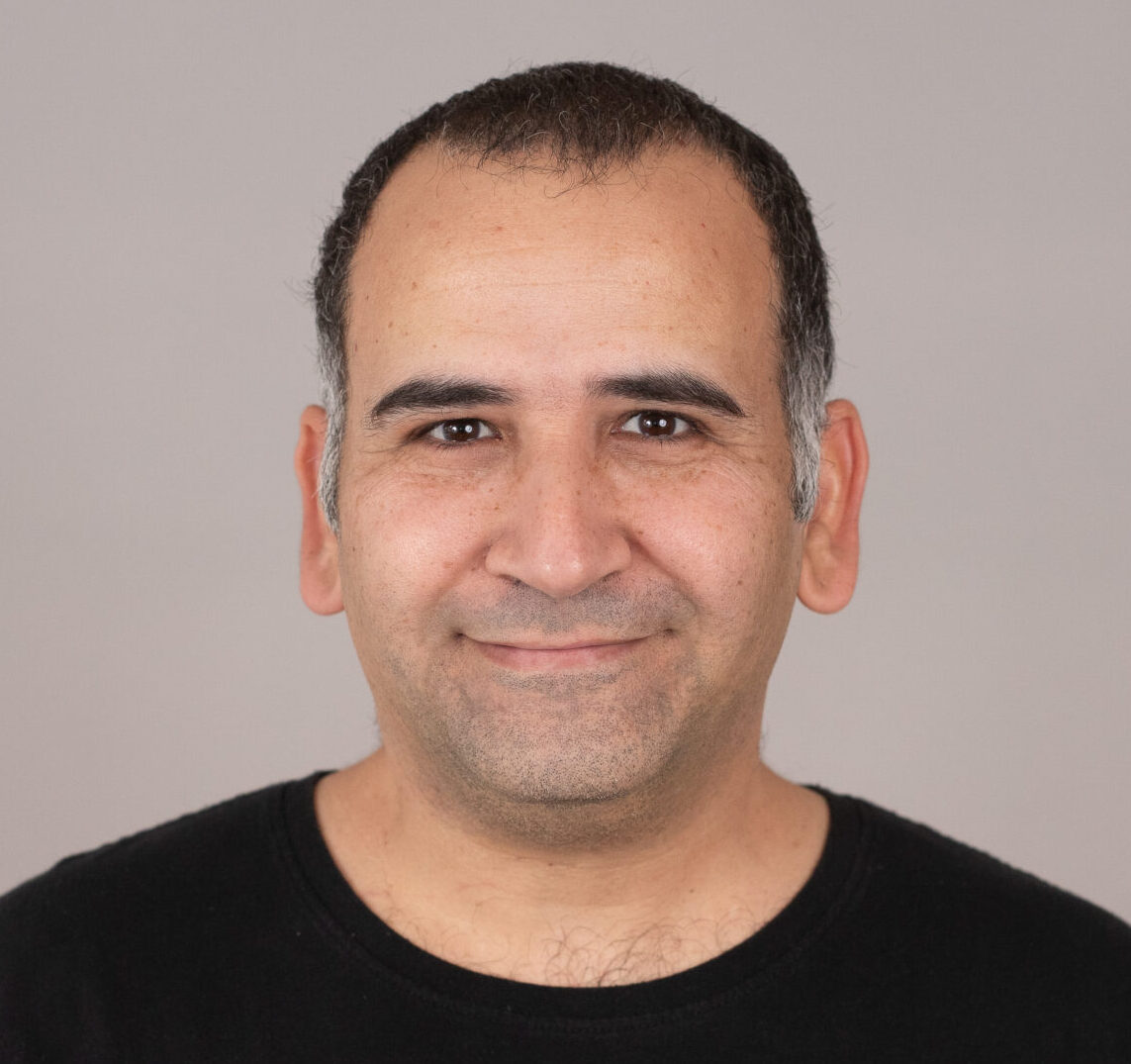
Yes. Triangulation, or orchestration, is key because different methodologies are suited to different use cases. Lets go for a very straightforward use-case: starting a new advertising channel.
ID Attribution: attributes ad engagement to conversions using a last-click model, giving 100% credit to the final touchpoint before conversion. This method can lead to over-attribution, especially if the new channel overlaps with existing audiences, resulting in cannibalization where attributed conversions are not truly incremental.
SKAdNetwork (SKAN): similar to ID attribution but provides aggregated reporting at the campaign level, still following a last-touch wins approach. It doesn’t offer detailed user-level insights but is useful for understanding campaign-level performance.
Media Mix Modeling (MMM): measures the correlation between spend and performance using top-down econometric models. The main challenge with MMM for a new channel is the lack of historical data, requiring significant spend and time to gather enough data for accurate evaluation.
Incrementality: measures the actual change caused by the new channel. This method is highly valuable for assessing whether the new channel is truly driving additional conversions or simply shifting them from existing channels. It provides clear insights into the channel’s effectiveness.
Other use cases may favor different methods. For example: Creative A/B test would benefit from ID attribution — since the granularity of reporting is low, and the results are real time.
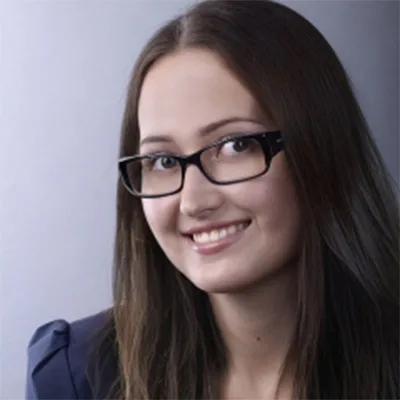
Which measurement models provide the most granular insights into user behavior and channel performance: MTA, MMM, or Incrementality? How do SKAN and other attribution methods compare?
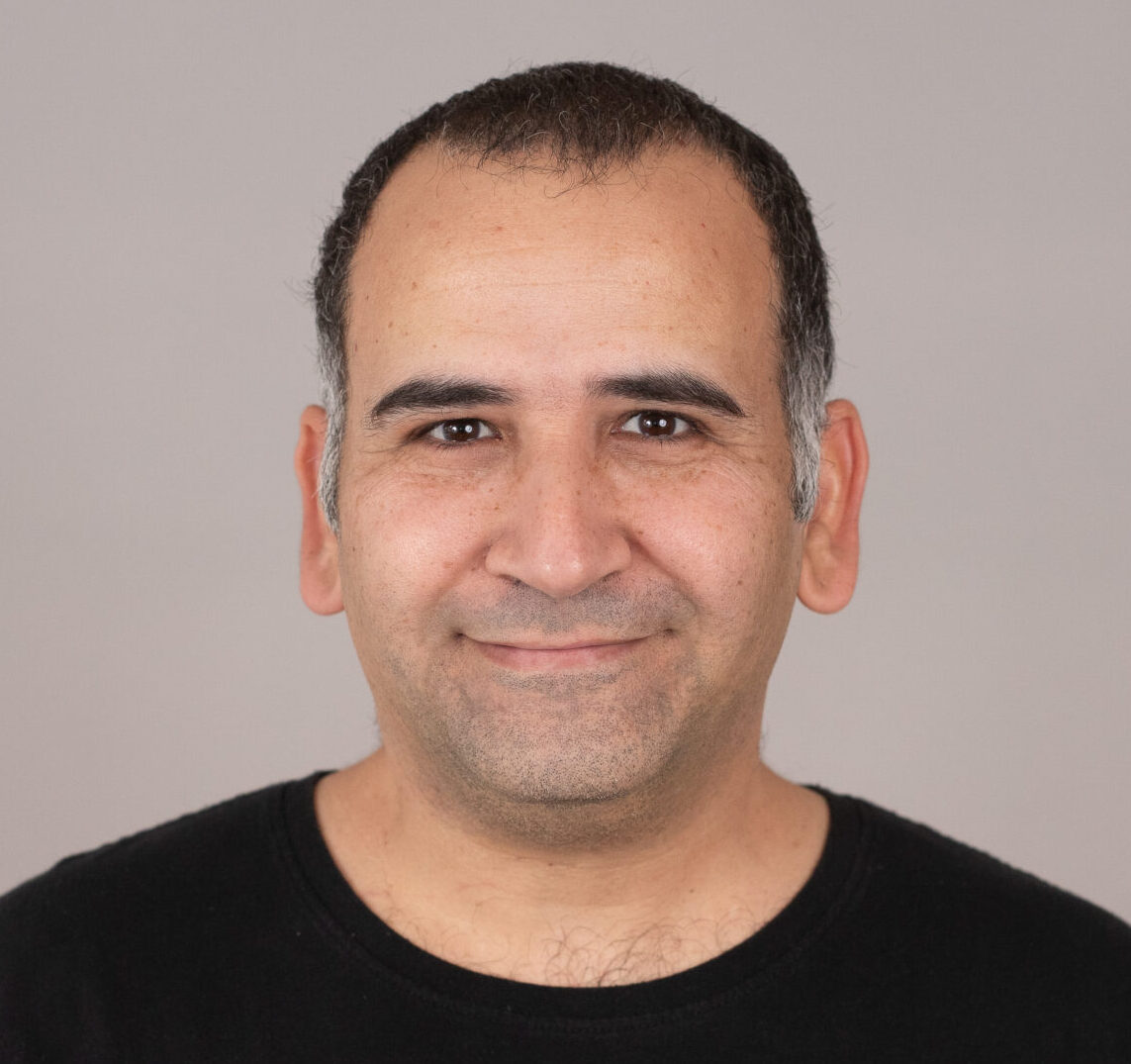
Among the methodologies, multi-touch attribution (MTA) offers the most granular insights. MTA tracks the user journey in detail, recording every interaction a user has with ads. However, MTA relies heavily on user data access, requiring consent and mechanisms such as cookies or IDFA. This reliance makes MTA less feasible in today’s privacy-conscious environment.
On the other hand, SKAdNetwork (SKAN) — soon to be Attribution Kit — provides campaign-level granularity within a 24-hour window. These methods attribute conversions to the last touchpoint a user interacted with before converting. While this approach is somewhat granular, it oversimplifies user journeys by crediting only the last touchpoint, ignoring prior interactions.
Media mix modeling (MMM) and incrementality measurement are less granular but serve different purposes. MMM focuses on high-level scenario planning and forecasting, offering insights at an aggregate level rather than individual user actions. Incrementality, while also less granular than MTA, provides retrospective analysis, identifying the actual impact of specific campaigns. It sits between MTA and MMM in terms of granularity.
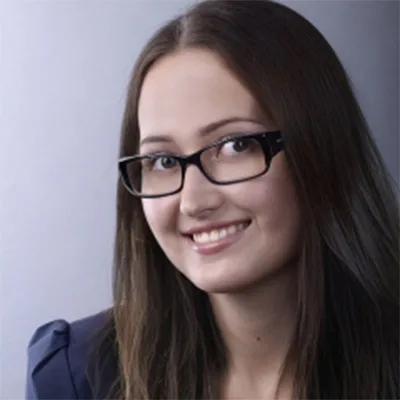
SKAN, MMM, and incrementality are all well-established concepts that are fundamentally about measurement. While each method serves different purposes, SKAN and MMM have gained more traction in the market. Why hasn’t incrementality caught on as much?
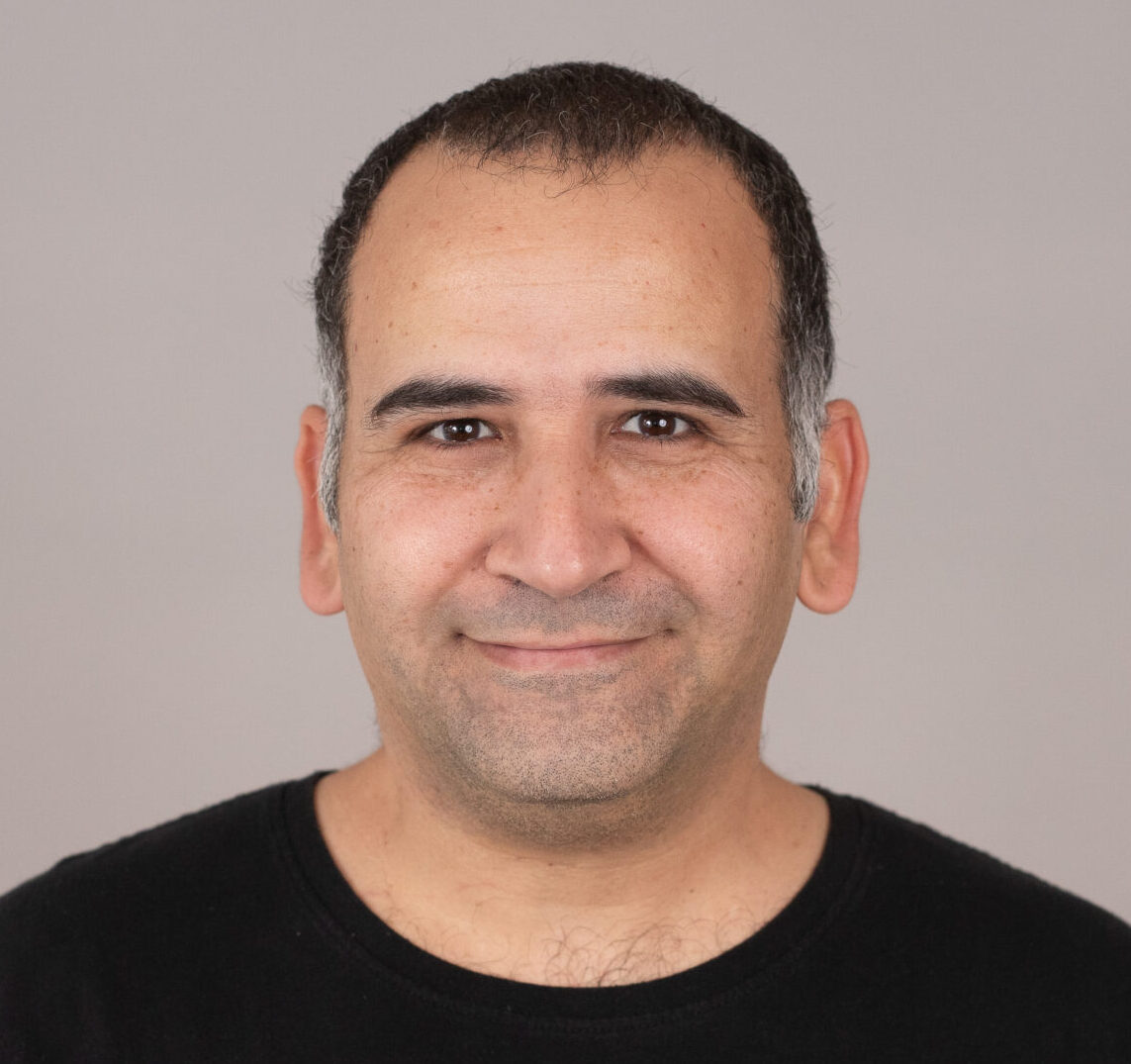
Incrementality, while a valuable measurement approach, hasn’t gained as much traction historically due to its practical challenges. Traditional incrementality testing required advertisers to pause their campaigns on specific platforms, then restart them and compare the differences in performance. This method necessitated a controlled environment where no other variables changed during the testing period, which is often impractical and disruptive for most businesses.
Media Mix Modeling (MMM) faced its own hurdles, being perceived as complex and not as deterministic as other methods, despite offering high-level forecasting and scenario planning. Privacy changes have led to a resurgence of both MMM and incrementality methods, but modern incrementality measurement has evolved significantly.
Our platform, for instance, offers a sophisticated approach to incrementality. It measures the incremental impact of advertising without needing to pause campaigns or conduct A/B testing. This advancement makes incrementality testing more feasible and appealing, leading to increased adoption across various industries. We have seen traction with notable clients from mobile companies to retailers, healthcare institutions, and entertainment giants.
The shift toward privacy-conscious measurement methods has revitalized interest in incrementality, especially with modern methodologies that simplify the process and reduce disruptions. This evolution addresses previous challenges, making incrementality a more practical and attractive option for today’s advertisers.
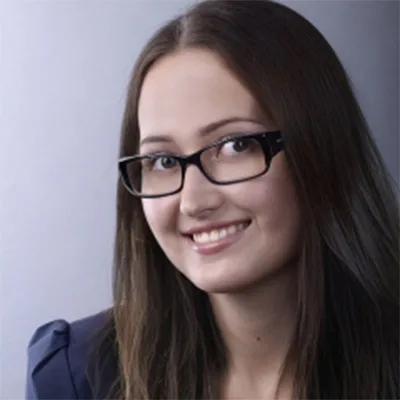
What does incrementality mean for brand advertising and for SSPs/DSPs that work with brand advertising?
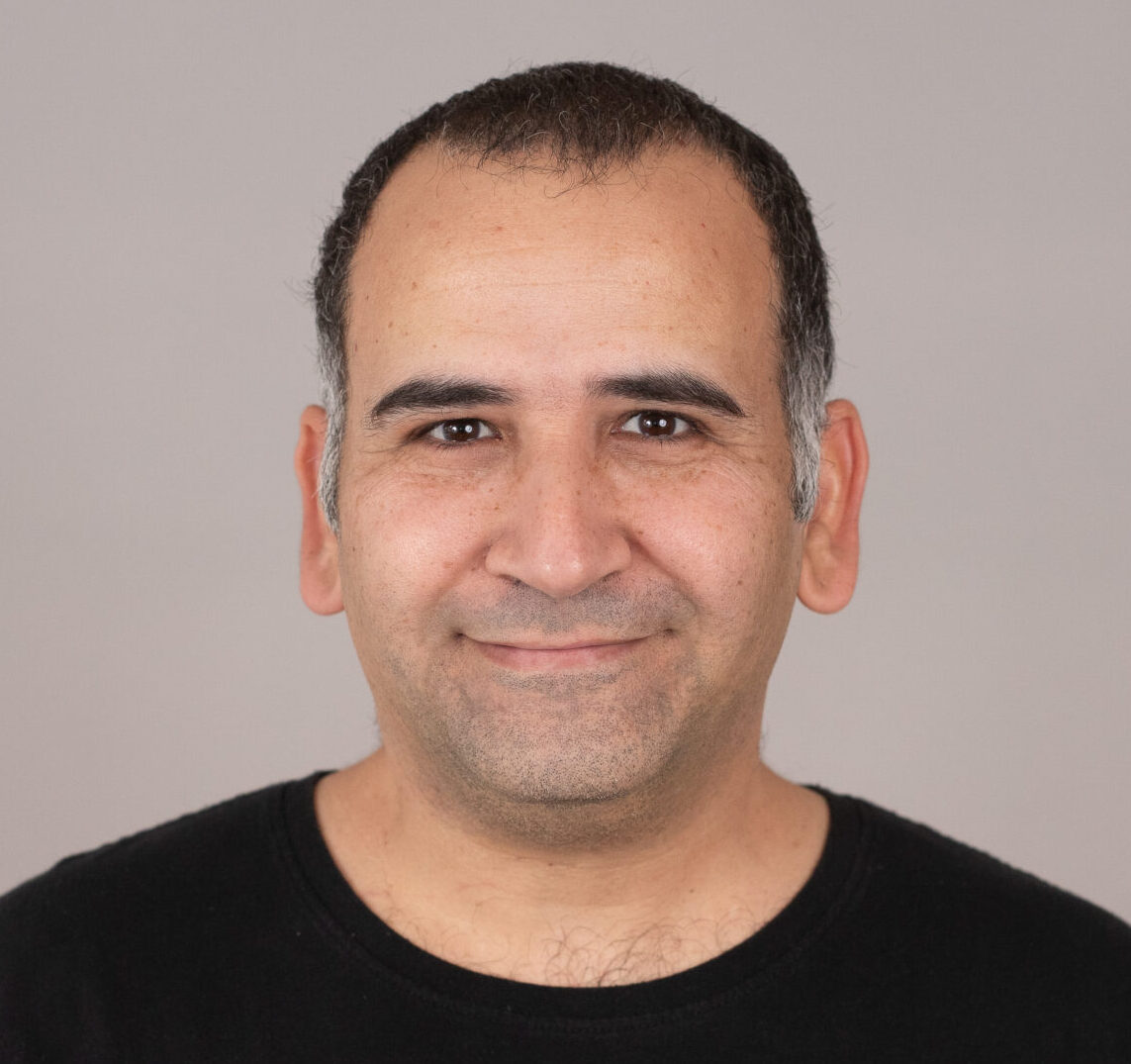
Every dollar spent on marketing needs to yield some outcome. Even luxury brands have specific objectives behind their advertising efforts. The main difference between brand and performance marketing lies in their trackability. Performance marketing, with its direct response elements, is inherently trackable. Brand marketing, on the other hand, focuses more on inspiration and holistic messaging. Everything is measurable. Not everything is trackable.
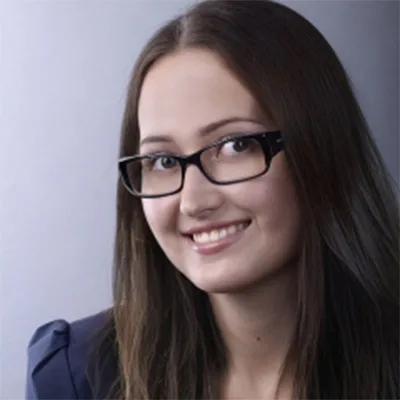
What trends do you foresee in the future of mobile app marketing measurement and attribution?
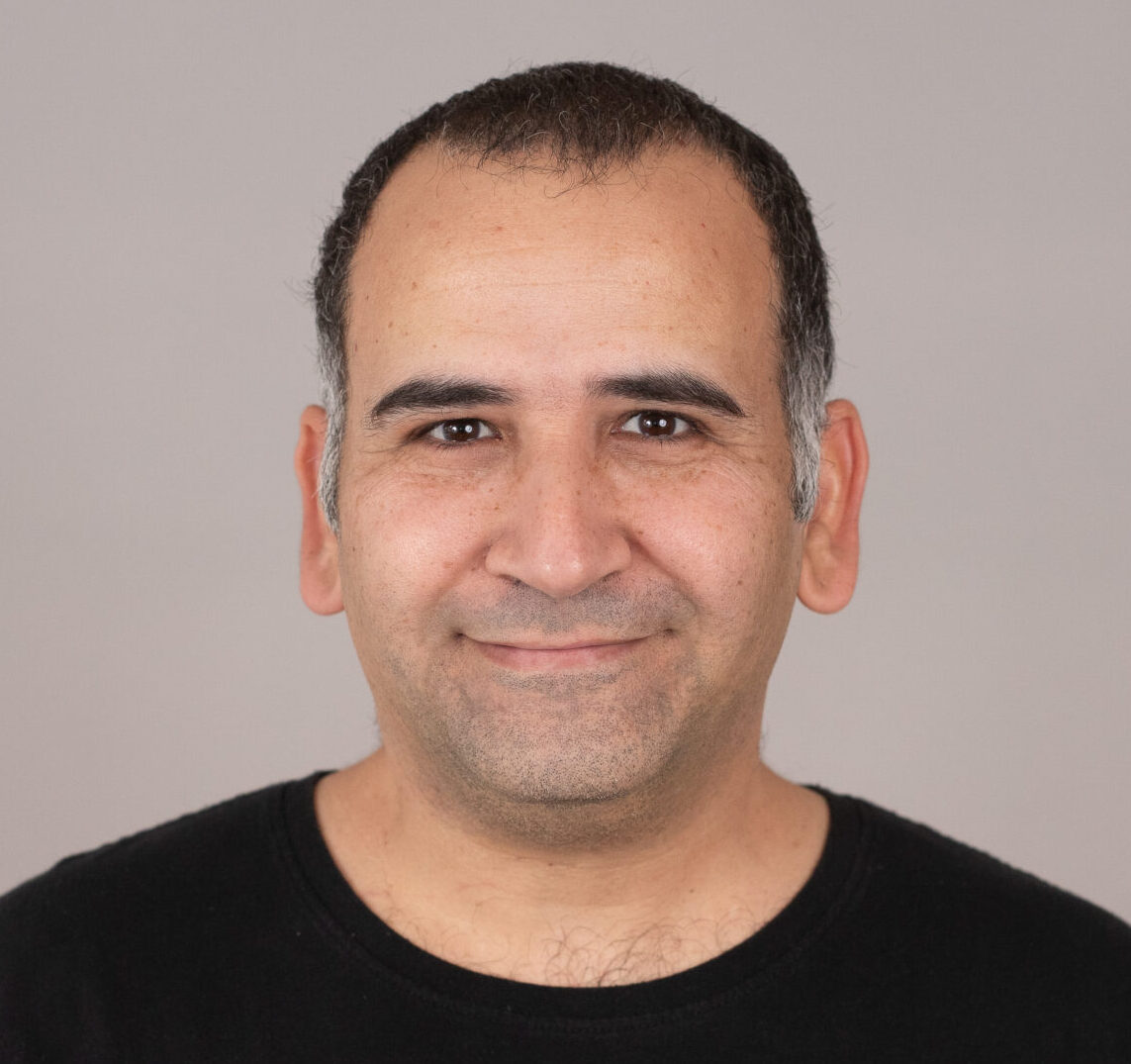
Algorithmic attribution is emerging as a dominant method, particularly due to shifts in privacy policies like Apple’s App Tracking Transparency (ATT). Platforms like Meta are increasingly relying on their vast first-party data and aggregated postbacks from SKAdNetwork to model conversions.
Platforms are using advanced algorithms to predict conversions based on user behaviors and historical data. This allows them to optimize targeting and deliver more accurate conversion estimates.
Algorithmic attribution often results in discrepancies with traditional attribution methods like MMPs (Mobile Measurement Partners), as platforms prioritize their own modeled data over third-party attribution reports.
The complete deprecation of ID attribution in favor of aggregated methodologies like SKAN and Google’s privacy-compliant methodology.
With the deprecation of IDFA and similar identifiers, the era of tracking individual users without consent is coming to an end. Privacy-compliant methodologies like Apple’s SKAdNetwork and Google’s Privacy Sandbox are gaining prominence.
Platforms are moving away from user-level data toward aggregated data methodologies that preserve user privacy while still providing actionable insights for advertisers. Even IP address tracking is becoming less reliable due to its dynamic nature and lack of persistence across different devices and locations.
Rise of incrementality as the primary measurement method. Incrementality measurement is gaining traction as advertisers seek more reliable metrics amidst the shift away from user-level tracking. Incrementality focuses on measuring the true lift in outcomes that can be attributed directly to marketing activities, regardless of the attribution method used. Modern incrementality methods allow for continuous optimization and testing without the need for drastic pauses in advertising, making them more suitable for dynamic marketing environments.
The future of mobile app marketing measurement and attribution is moving towards more sophisticated, privacy-conscious methodologies like algorithmic attribution and incrementality. While algorithmic attribution leverages platforms’ proprietary data to model conversions accurately, incrementality provides direct insights into campaign effectiveness without relying heavily on user-level tracking. MMM, while highly customizable, faces challenges in adoption due to its complexity and the increasing preference for real-time insights.
As the industry continues to adapt to privacy regulations and technological advancements, marketers will need to adopt a mix of measurement approaches tailored to their specific goals and operational needs. By leveraging a combination of these methodologies, marketers can make more informed and strategic decisions, optimizing their campaigns effectively across multiple dimensions.