Contextual isn’t new, but advancements will solve marketers’ headaches
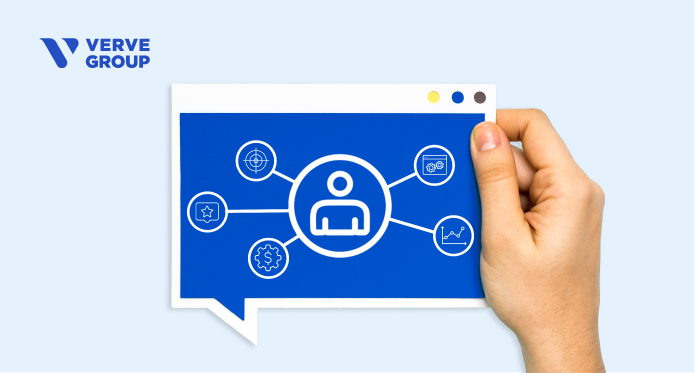
EDITOR’S NOTE: This article originally appeared in AdvertisingWeek. It was the rise of behavioral targeting, which tracks users’ online activities and personal data, that saw contextual advertising dethroned as the industry’s default targeting method. Fast forward to today and behavioral targeting is facing serious limitations, while significant developments in contextual are bringing this approach back to the fore. Here’s why . . . In the ever-evolving digital advertising landscape, marketers will face periodic challenges in effectively reaching and engaging their target audiences. Privacy is the latest challenge – and it’s a big one. The focus on privacy began in 2018 with the introduction of the General Data Protection Regulation (GDPR) in Europe. This was followed by similar initiatives worldwide, such as Apple’s App Tracking Transparency, Android’s Privacy Sandbox and Google’s upcoming phasing out of third-party tracking cookies on Chrome by the second half of 2024; beginning for 1% of users as early as the first quarter of 2024. Having to navigate restrictions that govern the collection and use of consumer data has left marketers frantically searching for alternative approaches. The advertising industry must go back to the drawing board, and the easiest way to start is by revisiting existing approaches that offer compliant targeting at scale. Contextual is emerging again as the dominant solution for marketers. The drawbacks of conventional approaches Contextual marketing revolves around understanding the environment where an ad appears and tailoring the content based on the relevant factors surrounding the user’s browsing experience. This approach leverages factors such as website content, keywords, location, and device type to deliver relevant and timely ads to users. However, recent research by TPA Digital has found some contextual solutions fall short of meeting their basic campaign goals. Advertisers often find themselves wasting significant spend on ads that fail to reach their target audiences. Inaccurate keyword analysis, limited contextual understanding, and insufficient real-time optimization are contributing to suboptimal campaign performance. The other issue is the growing problem of next-generation “content farms” created to hijack programmatic advertising revenue. An internet trust tool, NewsGuard, is tracking an increasing number of spammy, AI-generated news sites. They expect that generative AI will cause more of the estimated $2.6 billion of advertising revenue per year unintentionally funneled toward misinformation news sites. With this boom in the number of irrelevant, misleading and valueless articles published daily, advertisers now find themselves between a rock and a hard place when it comes to getting quality results from ad spend using conventional contextual targeting. AI-optimized contextual tools offer better targeting The good news is that machine learning, natural language processing, and data analysis are driving innovation in contextual targeting. These new technologies enable advertisers to analyze and interpret vast amounts of data to deliver highly targeted ads to the right audience. By considering the context of where an ad appears, marketers can target the user’s immediate needs and interests with specific messaging, leading to improved engagement and conversion rates. Through these advancements advertisers are able to reach consumers with accuracy and speed, maximizing the chances of engaging them in meaningful ways. Advertisers can also ensure they are able to deliver the personalized and engaging experiences consumers expect. For example, natural language processing technology is enabling hyper-accurate cohort targeting capabilities. There is also the potential for contextual solutions to “understand” and mine content beyond text content, such as brand audio, videos, and apps. Models are also being built on contextual capabilities that understand affinity and match content with the open web. Contextual empowers marketers to leverage their most valuable currency — attention. By moving away from clicks towards attention and reaching consumers in the moments that matter to them, they can fine-tune campaigns at any time towards their desired metrics – and ultimately improve outcomes. This real-time contextual analysis and AI-driven optimization means brands can advertise alongside highly relevant, fresh content, at the precise moment that it is attracting large audiences. As with many evolutionary matters, change can be painful, but also necessary. As the industry is pushed relentlessly towards the edge of the third-party cookie tracking precipice, innovations in contextual are ensuring that marketers can meet and engage with their audiences in the new privacy-first world.
The Media Trade Upgrade: An invitation to zero-party data and first-party data
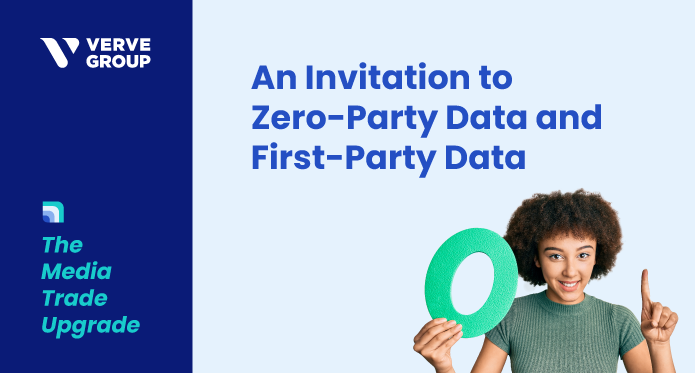
Welcome to the third edition of The Media Trade Upgrade, where programmatic traders can get essential info to stay ahead in ad tech – all in quick, bite-sized pieces. Consider today’s blog post your invitation to an exciting party . . . of zero-party and first-party data. We’ll quickly recap differences of the different types of data, all the way from the definition of zero-party to third-party data. (Don’t worry – there’s no such thing as fourth-party data . . . yet!) Then, we’ll dig into how programmatic media traders can use zero-party data alongside first-party data for media planning that crushes campaign goals. Countdown: From third-party to zero-party Triple Threat: Third-party data Third-party data describes how an entity without a direct relationship with the end-user collects that user’s data. Aggregators can obtain data from multiple sources to offer a broad view of users when combined with third-party inputs. This data can be stitched together across various inputs to help create a broader picture of a user. Third-party data works alongside first-party data to improve targeting lists. Determining if third-party data was legally collected is challenging with changing privacy regulations and user data control. Bridging the Gap: Second-party data Second-party data occurs when one entity shares data about its users with a trusted partner. The data quality and accuracy is high, since it was originally one company’s first-party data. Second-party data is also available for purchase on certain data marketplaces. Publishers may also sell information to marketers, such as an ecommerce platform selling purchase history or customer loyalty data to an advertiser. The Gold Standard: First-party data First-party data is information collected from a company’s customers or audience, such as profile information, website purchases, or loyalty programs. This type of data is given freely and verified for accuracy, making it highly valuable. Take a user who creates an account to access free content on a news site. Any personal information they provide directly to the publisher, such as their email, gender, or birth date, counts as first-party data. Similarly, purchase history is another key example of first-party data. Fast fact: 78% of businesses consider first-party data as the most valuable source of data for delivering personalization to audiences. The Unsung Hero: Zero-party data Zero-party data refers to information that users proactively and willingly share with brands and advertisers, typically through direct engagement such as surveys, polls, or preference centers. Unlike first-party data, which is collected through user interactions with a brand’s website or app, zero-party data is explicitly provided by the user, giving them even more control over their personal information. Benefits of zero-party data in advertising and media planning The impact of zero-party data on campaign planning is significant for several reasons: Better user experience Zero-party data often includes valuable insights into a user’s preferences, needs, and interests. This information allows advertisers to create more targeted and personalized campaigns, leading to increased engagement and conversion rates. In 2022, 62% of consumers said a brand would lose their loyalty if the brand delivers an un-personalized user experience, up nearly 20% from 2021. Enhanced trust and transparency By collecting zero-party data, brands can demonstrate their commitment to privacy and transparency. For example, a hair-care brand might use a quiz to create a custom shampoo formula tailored to the user. The quiz should be very explicit in communicating that the user’s input (a.k.a., zero-party data) will only be used to provide personalized product, content, or marketing experiences. This kind of transparency can help build trust between the brand and its audience, fostering long-term relationships and customer loyalty. Compliance with privacy regulations With the increasing focus on data privacy and the introduction of stricter regulations like GDPR and CCPA, using zero-party data can help agencies and brands ensure compliance. Since users willingly share zero-party information, there is less risk of violating privacy laws compared to relying on second- or third-party data sources. Reduced reliance on third-party data As browsers phase out third-party cookies and privacy concerns grow, advertisers need alternative data sources for targeting and personalization. Zero-party data offers a more reliable and sustainable solution, allowing brands to maintain effective marketing strategies despite changes. To incorporate zero-party data into campaign planning, media buyers at agencies should collaborate with their clients to create engaging experiences that encourage users to share their information. This may include interactive quizzes, polls, or preference centers that capture user insights while providing value in return, such as personalized recommendations or exclusive offers. By collecting zero-party data that can power future campaigns, advertisers can drive better results while respecting user privacy and fostering trust. Considerations Before RSVPing to the Zero-Party Parting Thoughts Data, privacy, and identity are top priorities in ad tech. Explore more in our in-depth eBook, Identity: Decoded, which unpacks the intricacies of identity for marketers, consumers, and the supply side of advertising. Follow along on LinkedIn, or subscribe to our newsletter below!
Now that publishers and advertisers are using ATOM, what have we learned — and what lies ahead?
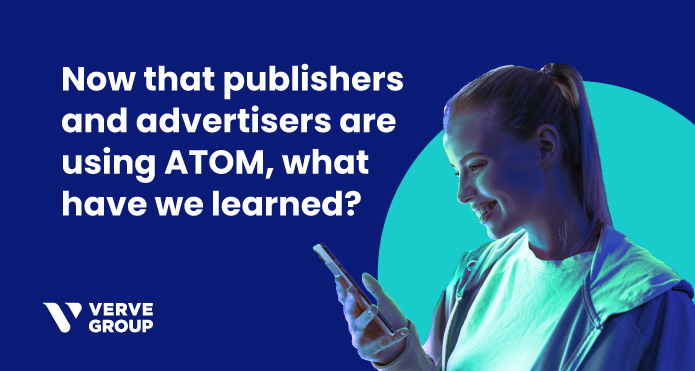
In 2021, Verve launched Anonymized Targeting on Mobile — ATOM. In response to GDPR and Apple’s new privacy strategy on iOS, ATOM was designed with the intent of providing a mobile-first advertising solution focused on machine-modeled behavioral and first-party contextual data. With ATOM, publishers can continue to show relevant ads to their users and offer advertisers to accurately reach their target audiences without IDFA. Now that ATOM has moved on from being our baby to being an ad-tech toddler, we have already recognized several accomplishments, milestones, perspectives and learnings. Mindsets are changing While Google recently announced (again) that cookies won’t crumble just yet, ATOM has proven to publishers and advertisers, alike, the necessity to adapt to a world without identifiers. Consumers have more control, choosing what kind of data is collected and for which purposes–not only under GDPR standards, but if they use an iOS device, for global standardization. Not using IDs for addressing the right audiences is now considered common practice by most advertisers. This is necessary, as 72% of iOS traffic hitting Verve’s mobile ad exchange is currently sent without an ID. Budgets are shifting While acceptance and adoption of ID-less solutions has surged, some brands have chosen the “easy route” by shifting budgets to Android. By nature, brands operating in verticals like automotive with little access to their own first-party data will rely on third parties to fill that gap (see our Media Planner’s Strategy Guide for more details). However, chasing IDs is not going to last in the long run, and most brands might not even need targeting granularity down to a specific user. Yet, maintaining addressability remains key. And alternative solutions that offer solutions at scale for segment- or cohort-based targeting such as ATOM will deliver the same — if not better — results for brand campaigns. In addition to that, these new approaches also offer improvements to other factors such as taking into consideration the environments in which brands place their ads, as well. Brand safety is a big concern with modern day marketing — one which Verve’s Moments.AI™ tackles by identifying threats and qualifying content in real time. Performance measurement is calling Now that advertisers have adopted alternative targeting strategies, voices for measurement and attribution without IDs are becoming louder. How can ad performance be measured without identifiers? There are a variety of ID-less measurement options to consider. – Media Mix Modeling Media mix modeling is a statistical method based on an extensive set of historical data. This method is great for forecasting, but has its weakness when it comes to measuring the impact of advertising for a product launch when no historical data has been recorded. To inform media mix modeling, campaigns are often turned off entirely to arrive at a baseline for measurement. However, this practice of turning off any marketing activity entirely is often not feasible for advertisers. – Incrementality Incrementality studies can range from “simple” A/B testing to testing by geography (e.g., promoting a product launch in one region while letting it run by itself in another). These options, however, become complex when many different messages are being tested in parallel.Marketing impact can also be measured without identifiers in consumer surveys or panels. This method can grant insights into consumer demographics, share of voice, purchase intent, and more. However, results rely on active survey or panel participation. An automated algorithmic based approach to incrementality is casual inference. By adding all possible factors to the equation, these models can granularly analyze which marketing activity leads to an increase in conversion results, and which marketing practice might lead to cannibalization. – Cohort-based measurement. Cohort-based measurement finds its application with solutions such as data clean rooms or contextual advertising. While data-clean rooms bundle different advertisers’ and publishers’ user data within a black box, any participant can easily send queries to retrieve cohort-based insights. At Verve, we create lookalike cohorts for traditional ad campaigns, based on the context into which an ad is embedded. By taking into consideration the environment in which high propensity audiences are navigating, we can add the brand safety factor back into the picture. Running a campaign for trailblazers for a major international tech company, we found a 300% uplift in CTR and a decrease of 96% in brand safety risk when targeting against contextual audiences, compared to traditional campaigns. An assortment of different levers, from brand safety risk to recency score help us optimize our campaigns, we can tailor any campaign towards the right target audiences. And we are not stopping here. What’s next? Verve sees a world without identifiers as being the norm instead of the exception. Above all else, the people who comprise Verve are focused on sharing new and exciting ways to show where and how a world without identifiers will span across various operating systems and environments to enable anonymized behavioral targeting and measurement. If you are interested in learning more about our contextual and ID-less capabilities, reach out to us.